Building a successful new product is prohibitively costly and complex for non-tech founders and enterprises.
High Cost
Engineering costly and recruitment difficult
Limited Knowledge
Lack of lean and agile startup processes
Poor Alignment
Agencies not aligned with business goals
We assemble thoughtful cross-functional teams at an unbeatable quality to price ratio.
Business Alignment
Align with the business plan and seek product market fit.
Talented Teams
Efficient teams that prioritize collaboration and product validation.
Agile & Lean
Proven methodologies to optimize activity and maximize efficiency.
User Validation
Systematic approach to user testing, analysis and iteration.
Team
Business alignment and team collaboration allow us to deliver high quality products and a delightful experience for our clients.
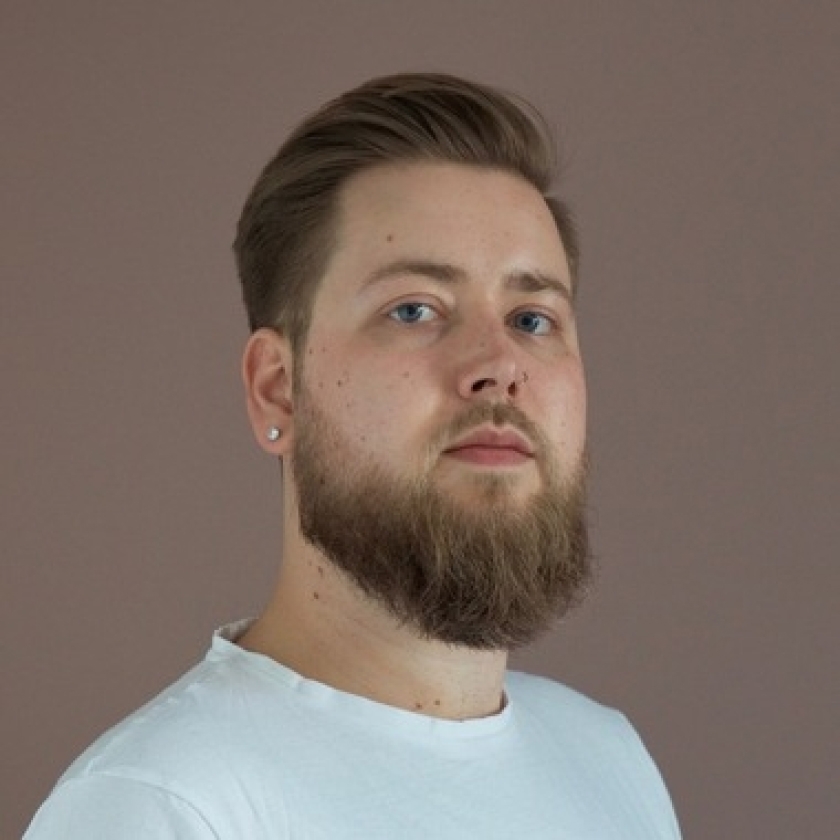
SPahmiule lA mLoeuwre
Founder / Product
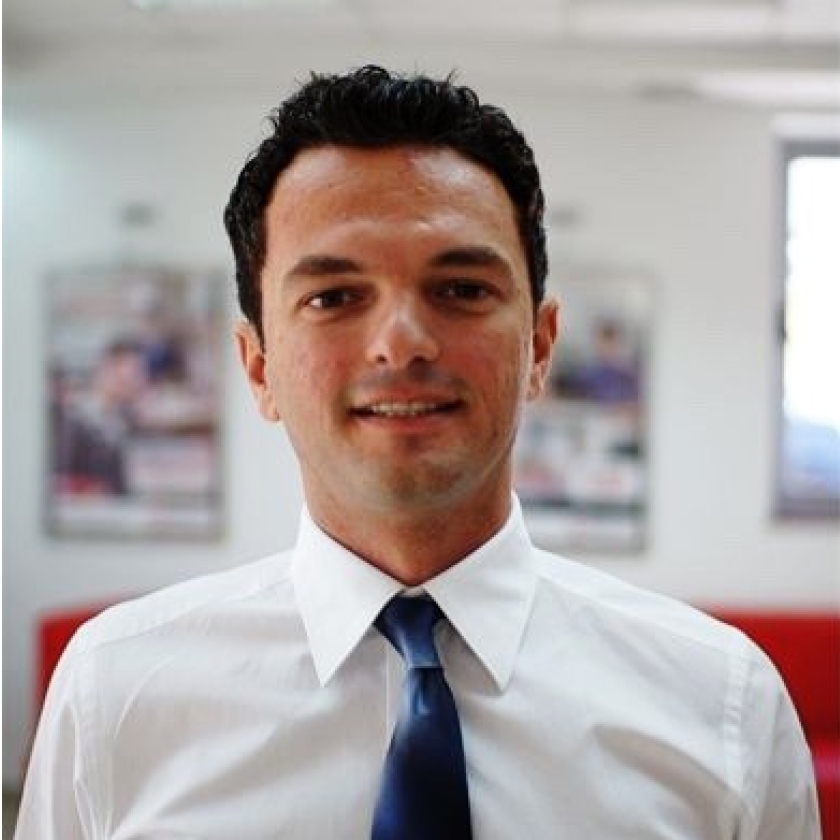
Arjan Ymeri
Operations
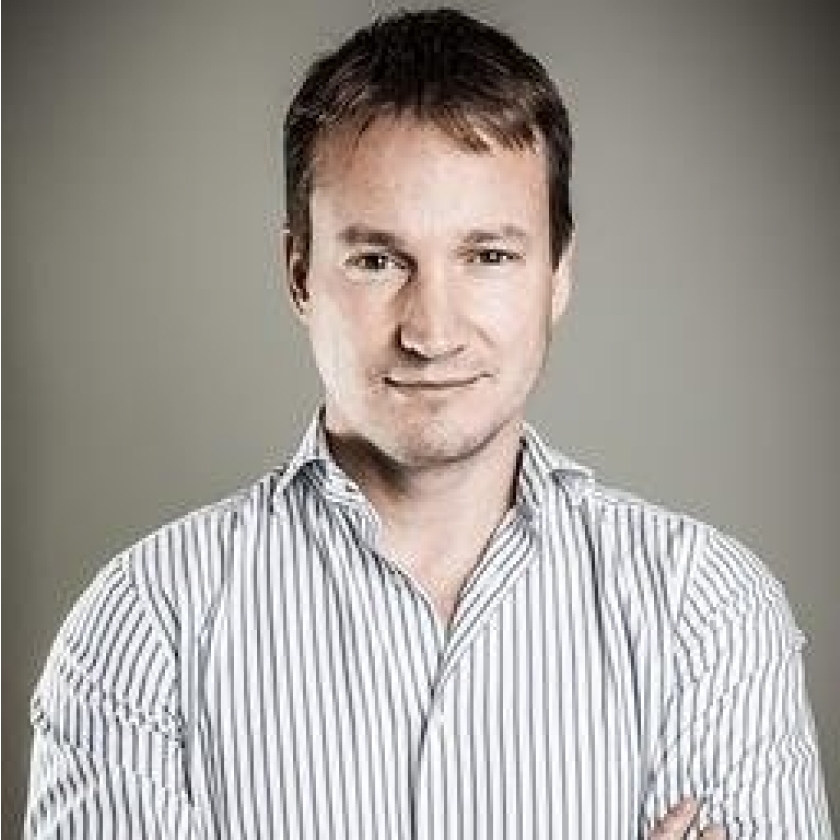
Arthur Holcombe
Business Development
How We Deliver
Scoping
Each project is led by a senior product designer and technical architect.
Building
We set up in-house cross functional teams that align with your requirements.
Operating
After you launch, we can maintain your project, or hand it over to you.
We build world–class products from zero.
We can handle the full end-to-end delivery, or support specific phases of your product development.
Scope Definition
Understand how it will work, and what it offers. Define the features and characteristics of the product.
Customer Interviews
Conduct market research to understand user needs and guide the product development.
UX & UI Design
Design an engaging product based on research and business requirements.
Prototyping & User Testing
Design prototypes with the purpose of user testing the product and gathering feedback.
QA & Testing
Run tests to report if the product is operating as intended.
Software Development
Provide elegant software solutions that are modern, secure, performative, and scalable.
Data Analytics
Track how users are interacting with your product. Turn data into business insights.
Early User Acquisition
Help you acquire early users quickly and cheaply achieving PMF and strong unit economics.
DevOps
Increase reliability and minimize service disruption. Continue to build, test and release software.
Let’s talk!
Fill out the form to start a conversation with us.